Accuracy of the Q-matrix-validation algorithm and sensitivity of model fit indices toward different types of Q-matrix misspecification for diagnostic classification models (DiaFit)
Diagnostic classification models (DCMs) are confirmatory multidimensional latent-variable models providing meaningful multivariate proficiency profiles. For the estimation of DCMs the specification of attributes involved in the solution process of items in a Q-matrix is required. Although various absolute and relative model fit indices have been proposed for DCMs, evaluating model fit of DCMs remains challenging. Another approach to evaluate the accuracy of DCMs is the Q-matrix validation method. In the project DiaFit a complex simulation study and an application study will be conducted. In the simulation the sensitivity of different absolute and relative model fit measures (e.g. AIC, BIC, MAD, RMSEA) to three different types of misspecified Q-matrices (underspecified, overspecified, balanced) will be investigated. Moreover, the robustness of Q-matrix validation algorithm will be addressed. In the simulation the number of respondents, attributes, and items will be varied, among other factors. The results will essentially extend the state of the art in model fit assessment and robustness of the Q-matrix validation algorithm and enable recommendations for the assessment of the model fit of DCMs in applied settings. The results of the simulation will be applied in a secondary analysis to two different datasets on arithmetic skills in elementary school that have already been collected. This application study will illustrate how different model fit indices and the Q-matrix validation method can be used in practical applications when the true data-generating Q-matrix is unknown.
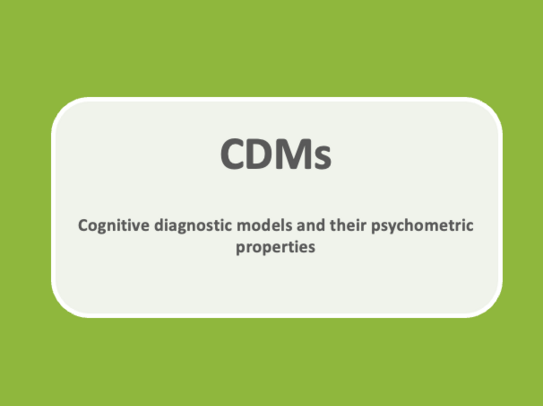
Akkuratheit des Q-Matrix-Validierungsalgorithmus und Sensitivität der Modellfit-Indizes für verschiedene Arten der Q-Matrix-Fehlspezifikation für kognitive Diagnosemodelle (DiaFit)
Kognitive Diagnosemodelle (DCMs) sind konfirmatorische multidimensionale latente Klassenmodelle, die aussagekräftige multivariate Leistungsprofile ermöglichen. Für Schätzung von DCMs ist die Spezifikation von Attributen in einer Q-Matrix erforderlich. Obwohl verschiedene absolute und relative Modellfitindizes für DCMs vorgeschlagen wurden, bleibt die Beurteilung der Modellanpassung bei DCMs eine Herausforderung. Ein weiterer Ansatz zur Bewertung der Korrektheit der DCMs ist die Q-Matrix-Validierungsmethode. Im Rahmen des Projekts DiaFit werden eine komplexe Simulationsstudie und eine Anwendungsstudie durchgeführt. In der Simulation wird die Sensitivität verschiedener absoluter und relativer Modellfitmaße (z.B. AIC, BIC, MAD, RMSEA) für drei verschiedene Arten von fehlspezifizierten Q-Matrizen untersucht. Außerdem wird die Robustheit des Q-Matrix-Validierungsalgorithmus untersucht. In der Simulation werden u.a. die Anzahl der Befragten, Attribute und Items variiert. Die Ergebnisse werden den Wissensstand bezüglich der Beurteilung der Modellpassung und der Robustheit des Q-Matrix-Validierungsalgorithmus wesentlich erweitern und Empfehlungen für die Beurteilung der Modellfitindizes bei DCMs in der Praxis ermöglichen. Die Ergebnisse der Simulation werden in einer Sekundäranalyse auf zwei verschiedene Datensätze zu arithmetischen Fähigkeiten in der Grundschule angewandt, die bereits erhoben wurden. Diese Anwendungsstudie wird veranschaulichen, wie verschiedene Modellfitindizes und die Q-Matrix-Validierungsmethode eingesetzt werden können, wenn die wahre Q-Matrix unbekannt ist.
Projektleitung: Prof. Dr. Olga Kunina-Habenicht
Kooperationspartner: Dr. Philipp Sonnleiter, Universität Luxemburg
Finanzierung: Deutsche Forschungsgemeinschaft (DFG) (KU 3647/2-1)
Laufzeit: 3 Jahre
Ravand, H., Effatpanah, F., Kunina-Habenicht, O‑., & Madison, M. J. (2025). A didactic illustration of writing skill growth through a longitudinal diagnostic classification model. Frontiers in Psychology, 15. https://doi.org/10.3389/fpsyg.2024.1521808
Ravand, H., Effatpanah, F., Ma, W., de la Torre, J., Baghaei, P., & Kunina-Habenicht, O. (2024). Exploring Interrelationships Among L2 Writing Subskills: Insights from Cognitive Diagnostic Models. Applied Measurement in Education, 37(4), 329–355. https://doi.org/10.1080/08957347.2024.2424550
Kunina-Habenicht, O., Rupp, A. A., & Wilhelm, O. (2017). Incremental validity of multidimensional proficiency scores from diagnostic classification models: An illustration for elementary school mathematics. International Journal of Testing, 17(4), 277–301. doi:10.1080/15305058.2017.1291517
Kunina-Habenicht, O., Rupp, A. A., & Wilhelm, O. (2012). The impact of model misspecification on parameter estimation and item-fit assessment in log-linear diagnostic classification models. Journal of Educational Measurement, 49(1), 59–81. doi: 10.1111/j.1745-3984.2011.00160.x
Kunina-Habenicht, O., Rupp, A. A., & Wilhelm, O. (2009). A practical illustration of multidimensional diagnostic skills profiling: Comparing results from confirmatory factor analysis and diagnostic classification models. Studies in Educational Evaluation, 35(2), 64–70. doi: 10.1016/j.stueduc.2009.10.003
Kunina-Habenicht, O., Wilhelm, O., Matthes, F. & Rupp, A. A. (2010). Kognitive Diagnosemodelle: Theoretisches Potential und methodische Probleme. Zeitschrift für Pädagogik, Beiheft 56, 75–85.